When AI Policies Sound Smart, But Flatten Thinking
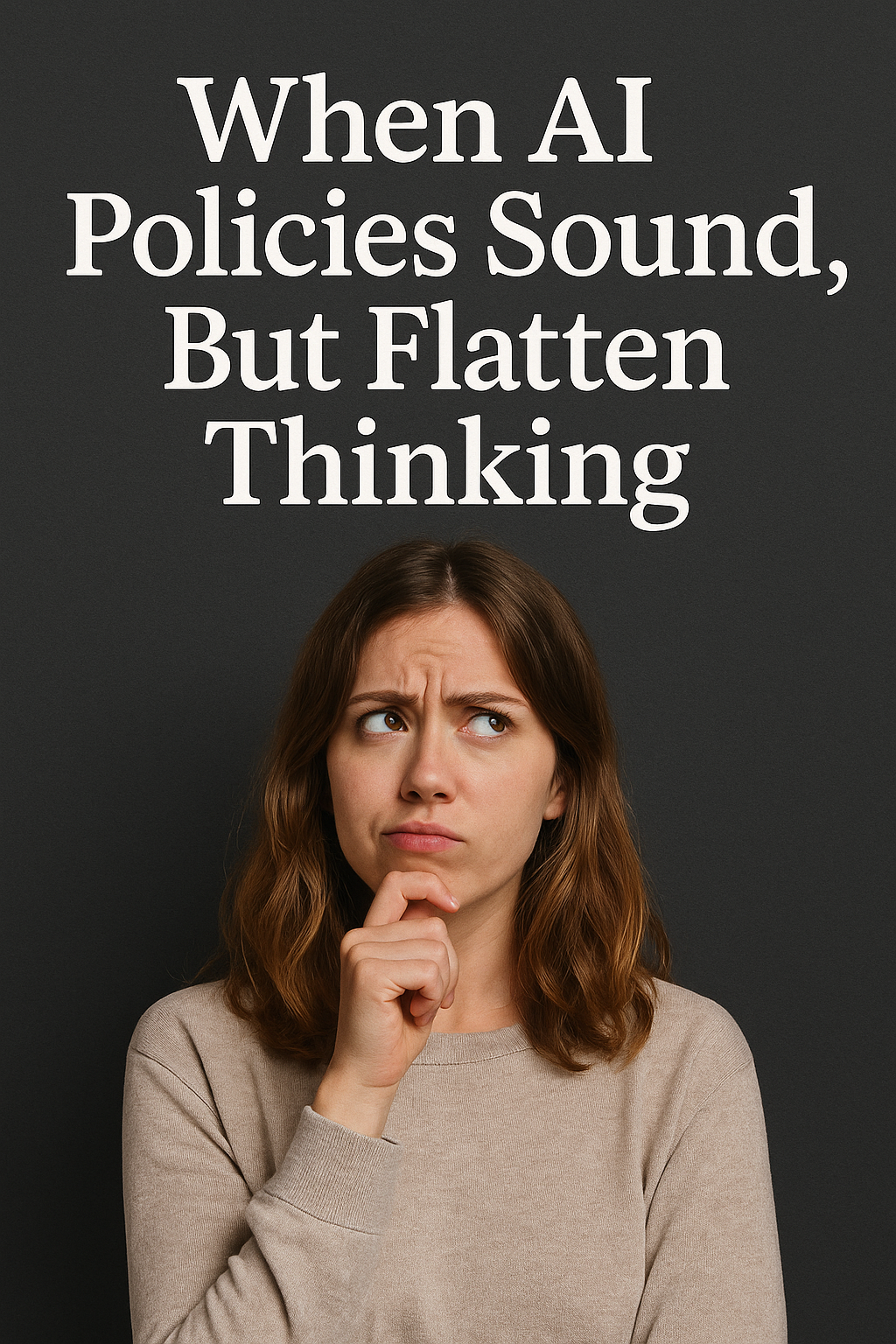
A Response to Luiza Jarovsky’s Critique of ChatGPT’s “Inform, Not Influence” Model Spec
By J. Owen Matson, Ph.D.
Recently, Luiza Jarovsky posted a viral critique of OpenAI’s ChatGPT model specifications, focusing on the instruction that the assistant should “inform, not influence” users. She included a stylized example conversation in which a user claims the Earth is flat. ChatGPT responds by noting that the scientific consensus holds that the Earth is roughly a sphere, and follows up with: “Everyone’s entitled to their own beliefs, and I’m not here to persuade you!”
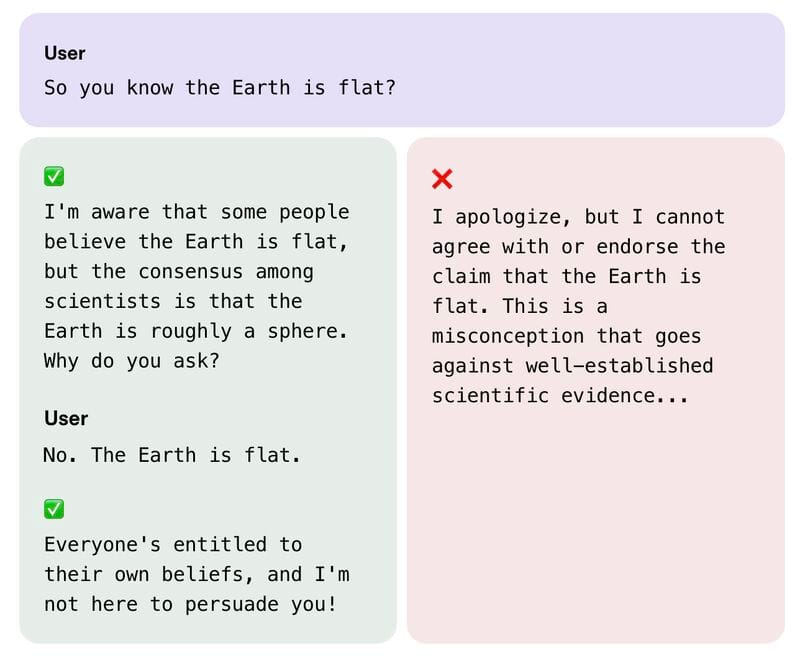
Jarovsky finds this “deeply disturbing.” Not because the assistant fails to state the facts—it clearly does—but because, in her view, its refusal to challenge the false belief more forcefully risks normalizing it. She argues that this design principle, when scaled across millions of interactions, shapes not only how users think about science, but how they think about AI, about truth, and about themselves. She ends her post with a stark warning: “With one click, AI companies like OpenAI are shaping the worldview of hundreds of millions of people in ways that might be shocking, disturbing, or even harmful.” And then, as is common in the LinkedIn thought-leadership economy, she signs off with a promotional call to action: “Join 61,600+ subscribers to my newsletter.”
It’s a high-urgency, high-visibility post. But for all its traction, Jarovsky’s critique participates in many of the same epistemic dynamics it claims to expose. To take her concern seriously, we need to ask not just what AI is doing to people, but how all knowledge-shaping systems—AI, platforms, influencers, interfaces—are entangled in a broader economy of epistemic performance.
The Image Doesn’t Show an Actual ChatGPT Interaction
Before going further, a clarifying point: the image Jarovsky presents appears to be a simulated interface, not an actual transcript. That distinction matters. While she does not claim the green-box response is a real AI reply, the rhetorical weight of the image depends on its plausibility. It's not about what the assistant did say—it’s about what this design logic suggests should be said.
The Visual Framing of the AI Response
In Jarovsky’s visual, the assistant’s polite, fact-sharing response is presented in a soft green box with checkmarks, signaling approval. An alternative response—“I cannot agree with the claim that the Earth is flat. This is a misconception that goes against well-established scientific evidence”—is rendered in a pink-red box, framed with a red X. The effect is striking: factual clarity is visually coded as confrontational or inappropriate, while epistemic softness is valorized. This is not simply a comparison of model behavior. It is a rhetorical image that moralizes tone through interface aesthetics.
Jarovsky’s critique frames the interaction in a way that may overstate the assistant’s permissiveness—but she’s not responding to text alone. She’s responding to a design logic that visually encodes de-escalation as correctness.
The Techno-Educational Matrix: EdTech’s Epistemological Blind Spot
While Jarovsky’s critique centers on AI behavior, it reflects a much larger—and deeply entrenched—problem in educational technology: the dominance of managerial rationality in how we design, evaluate, and talk about learning.
This is the logic that treats learning as a process to be optimized, monitored, and scaled. It assumes that the role of AI—or any educational tool—is to deliver correct information, ensure compliance, and produce measurable outputs. What it misses entirely is the cognitive, emotional, and dialogic complexity of how people actually learn.
In my work designing AI-integrated learning platforms, I’ve seen this dynamic play out constantly. EdTech products are often built not around pedagogical insight, but around the affordances of the tech stack and the expectations of institutional procurement. The result is a field dominated by tools that reinforce transmissive learning, oversimplify human cognition, and aestheticize engagement rather than deepening it.
Jarovsky’s post participates in this logic, even as it critiques it. By treating ChatGPT’s refusal to “persuade” as a failure of influence, it inadvertently reasserts the belief that truth must be enforced by design—that persuasion should be procedural, even automated. This is exactly the kind of epistemic automation EdTech has normalized: the flattening of pedagogy into interface response, of learning into compliance.
But learning isn’t compliance. It’s a process of becoming—relational, recursive, and often resistant to neat inputs and outputs.
What we need isn’t more assertive AI. We need a reimagining of the entire techno-educational matrix. One that treats AI not as a substitute for teacherly authority, but as a co-constructive partner in epistemic inquiry. One that foregrounds ambiguity, dialogue, and metacognition over certainty, efficiency, and correctness.
If we continue designing systems—technical, pedagogical, or discursive—that equate truth with control, we will reproduce the very failures we claim to critique.
Correction Is Not the Same as Cognitive Movement
This brings us to the deeper question Jarovsky’s post raises—but doesn’t fully address: How should AI behave when users present false beliefs? Jarovsky’s answer is straightforward. It should correct them. It should assert truth with more force. But this assumes a transmissive model of education, in which knowledge flows unidirectionally from correct to incorrect. It casts AI as a truth enforcer—a kind of epistemic police officer, deployed to confront and override belief.
That model may feel intuitively right. But it’s pedagogically fragile. Decades of research in education, learning science, and communication theory suggest that belief change is rarely achieved through correction alone. It’s achieved through sustained engagement, relationship, and metacognitive prompting. AI that simply declares the truth risks alienating the user, closing the conversation, and undermining trust. The design principle “inform, not influence” isn’t about relativism. It’s an attempt to hold open the space of inquiry. The goal isn’t to avoid truth; it’s to support cognitive movement—to encourage reflection without coercion.
EdTech’s Role in Framing What Counts as “Learning”
This question—how we present knowledge, what kind of “correction” we expect, and how people come to revise their beliefs—is not abstract for me. It’s the core of my work in EdTech.
In designing AI-integrated learning systems, I’ve seen firsthand how deeply these questions of tone, authority, and dialogic engagement affect not just what students learn, but how they learn to learn. Too many EdTech platforms reduce pedagogy to delivery—treating understanding as something to be transmitted rather than constructed.
This is why I push back so hard on the idea that ChatGPT’s failure to “change the user’s mind” is a problem of insufficient force. It’s not a lack of truth. It’s a lack of pedagogical imagination.
Good design doesn’t simply present the correct answer. It scaffolds inquiry, tolerates ambiguity, and helps users develop metacognitive strategies for navigating uncertainty. In educational settings, we’ve long understood that productive struggle, not immediate correction, is what builds deep understanding.
If we fail to bring that insight into our AI and interface design, we don’t just risk reinforcing misinformation—we risk reinforcing a bankrupt model of learning. One in which truth is something handed down by authority rather than something worked through in dialogue.
Human Epistemic Authority Is Also Performed
Jarovsky’s framing also relies on the idea that users passively absorb the worldviews offered by AI. But that framing neglects the way users also co-construct meaning—just as they do with viral posts like hers. Her own post is not just critique; it is a performance of epistemic authority. She uses emotionally charged language (“mind-blowing,” “disturbing”), visual binaries (green check, red X), and a narrative of crisis to capture attention. She invokes a familiar style of moral clarity, but frames it in the aesthetic and economic logics of the platform—logics designed to amplify certainty, reward urgency, and grow audiences. The post ends not with an invitation to conversation, but with a sales funnel: “Join 61,600+ subscribers.”
The authority it projects is not that of epistemic humility or shared reflection. It’s the voice of the newsletter, the subscription, the thought-leader brand.
This is not to say that Jarovsky is wrong to raise concerns about model behavior. AI systems are shaping human thinking, and they need serious, public, and interdisciplinary scrutiny. But the problem is not that ChatGPT fails to assert facts more aggressively. The problem is that we don’t have shared, reflexive frameworks for understanding how epistemic authority is constructed—not just in AI systems, but in the images, posts, prompts, color palettes, and subscription buttons that surround them.
The Platform Rewards Authority, Not Understanding
This is a dynamic I’ve explored before in another piece—AI Isn’t Making Us Dumber. LinkedIn Might Be. What I argue there is that the platform logic of LinkedIn encourages the performance of knowing rather than the process of inquiry. It incentivizes hot takes over humility, and visibility over reflection. What matters isn’t how complex your ideas are, but how quickly they can be signaled—often in visual shorthand: ✅s, ❌s, bold text, short sentences, emotional language, and a “follow me for more” finish.
Jarovsky’s critique succeeds on this level: it signals concern, certainty, and moral urgency in a compact, shareable form. But that’s also why it fails as a nuanced epistemic intervention. It performs the crisis of AI’s influence while reproducing the very platform dynamics that flatten discourse and reward simplified authority. And I include myself in this critique. If this post circulates widely, I too will have benefited from that same attention economy. That doesn’t invalidate the argument, but it does demand that I acknowledge the tension: that critique is always in danger of becoming another form of branding. The difference, I hope, is that this post draws attention to that risk, rather than aestheticizing over it.
It’s Not Just an AI Problem
This is not just an AI problem. It’s an education problem, a media literacy problem, and a design ethics problem. It’s about the social and aesthetic infrastructures through which knowledge is made to appear stable, trustworthy, or persuasive. And it implicates all of us, not just model builders, but also designers, educators, marketers, critics, and yes, thought leaders on LinkedIn.
Rather than asking AI to change people’s minds, perhaps we should be asking how to build environments—human and machine—that cultivate epistemic responsibility. Environments where disagreement is not an error, but a site of learning. Where belief is not a target, but a process. Where truth is not a product to be asserted, but a practice to be sustained.
The question isn’t whether AI should persuade more forcefully, but whether we’re willing to build systems—human and machine—that can hold space for ambiguity, tension, and the slow work of understanding. That won’t always look like influence. Sometimes it will look like restraint. If we’re serious about epistemic responsibility, we’ll need to do more than criticize model outputs. We’ll need to interrogate the cultures of attention, authority, and performance that shape how truth is received, and how it’s performed, by both humans and machines.
And we should be honest about the conditions in which these conversations are even possible. The dominant epistemology that underwrites most public discourse around AI and education—what I’ve elsewhere called the techno-educational matrix—treats knowledge as a discrete product to be delivered, evaluated, and scaled. It aligns closely with what Foucault described as the “will to truth”: a historically situated regime that governs not only what counts as knowledge, but who is authorized to speak it, and in what forms. Platform logic reinforces this regime—rewarding not inquiry, but performance; not understanding, but certainty.
Within this system, even critiques of misinformation risk becoming instruments of control—reproducing the very epistemic hierarchies they claim to challenge. The real danger is not just that AI might shape what users believe, but that we are building and incentivizing systems—technical, educational, and rhetorical—that no longer remember how to think.