Beyond Augmentation: Toward a Posthumanist Epistemology for AI and Education
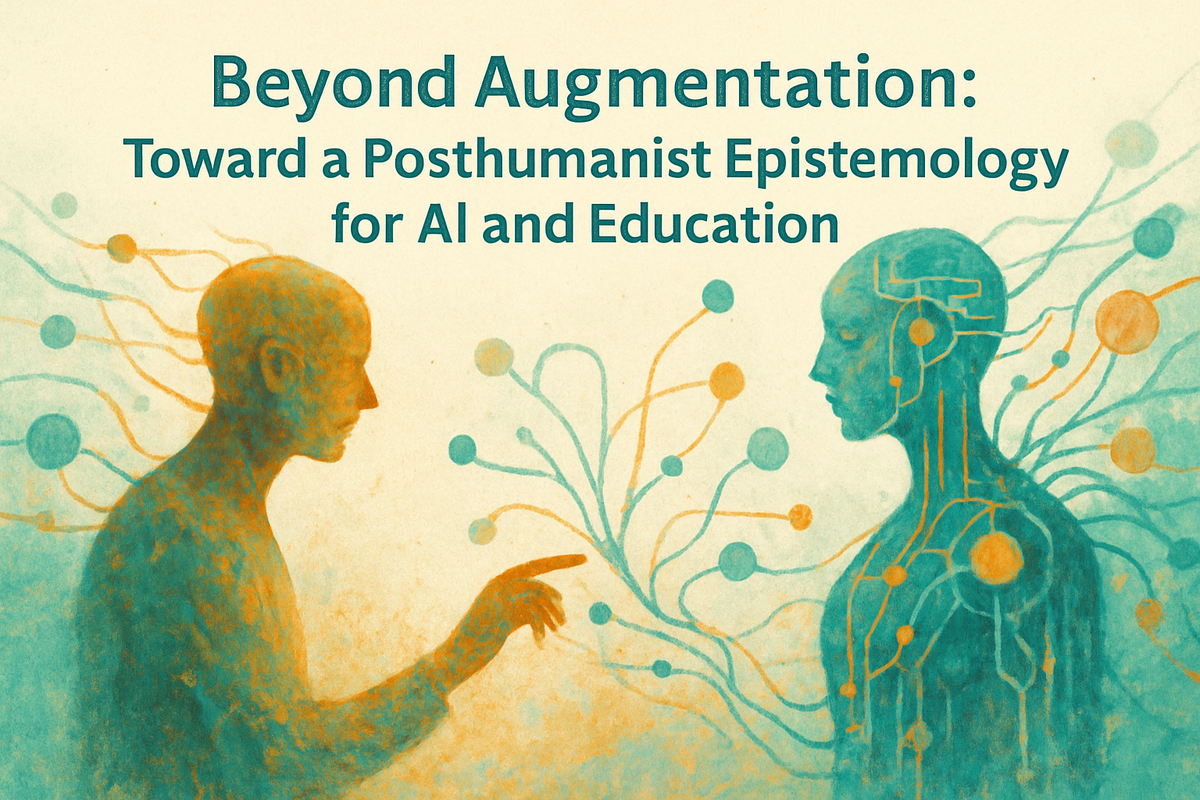
A Response to the Important Work of Chris Dede on AI in Education
J. Owen Matson, Ph.D.
This essay was written in response to Dede’s recent public invitation for feedback on his keynote lecture exploring the role of generative AI in shaping human creativity. In that talk—as in much of his recent work—Dede advocates for an educational paradigm in which AI is used to support and extend human capacities rather than replace them. I appreciate and respect this position, and my intention here is to take up Dede’s invitation in the spirit of constructive dialogue. While I share many of his concerns and commitments, I also aim to push the conversation further—questioning some of the epistemological assumptions that underlie even well-intentioned human-centered approaches to AI in education.
Chris Dede's recent post on LinkedIn shares powerful insights on the role of generative AI in augmenting human creativity.
I have long admired Chris Dede’s work and have followed his thinking on AI and education for years. His distinction between “reckoning” and “judgment” provides an essential framework for resisting reductive, automation-centered narratives in EdTech. I share his desire to foreground uniquely human capacities—ethical reasoning, contextual discernment, empathy—as central to the future of learning.
This essay argues that the dominant discourse around AI in education—even when well-meaning—remains trapped within a humanist epistemology that misrepresents how cognition unfolds in AI-human systems. Thinkers like Chris Dede, whose work I respect and engage closely, have done essential work to push back against automation-centered models by foregrounding human judgment, empathy, and creativity. Yet the very language of augmentation and partnership they rely on assumes a stable human subject whose cognitive authority is merely extended by AI. This framing cannot account for the distributed, emergent, and relational nature of thinking in AI-mediated environments. What is needed is not a defense of human uniqueness, but a reconfiguration of our epistemological assumptions—one that embraces a posthumanist understanding of learning as dialogic, unpredictable, and co-constructed across systems of human and machinic interaction.¹
The argument unfolds in six parts. I begin by examining how the language of augmentation and partnership in Dede’s recent public statements reflects a broader humanist commitment to cognitive sovereignty. From there, I contrast this with a posthumanist view of AI-human entanglement, where cognition is not extended but reconstituted. I then turn to personalization, arguing that even thoughtful models often fail to grant learners epistemic agency—treating them as data points to adapt to rather than co-participants in knowledge formation. This leads into a rethinking of the teacher’s role: not as epistemic authority, but as a relational steward. I then introduce the concept of dialogic cognition and the cognitive intraface as a new design paradigm for AI-human learning systems. Finally, I situate all of these arguments within the larger techno-educational matrix—the epistemological infrastructure that governs how we define, value, and design for learning itself.²
While Dede’s intervention offers an important counterpoint to dominant narratives of automation, it emerges within a broader discourse that increasingly frames AI in education through the language of enhancement: augmentation, support, partnership. These terms are appealing, especially when set against the specter of replacement. They suggest continuity, collaboration, and a reaffirmation of human centrality. But they also carry with them an epistemological assumption—often unexamined—that the human subject remains intact and coherent, merely improved by the presence of AI.
In particular, Dede’s remarks in his 2023 Harvard EdCast appearance, "Educating in a World of Artificial Intelligence," reiterate a familiar structure. He argues that AI should take on "lower-level teaching tasks" to free up teachers to do what "only humans can do": namely, provide judgment, ethical reasoning, and relational support.³ "We have to prepare students not just to use AI," he explains, "but to have a broader kind of intelligence that includes emotional and social skills." This framing envisions a tiered cognitive division of labor—AI handles reckoning, humans retain judgment. And while this protects against automation-as-replacement, it risks cementing a hierarchical model of cognition that fails to engage with how human and AI systems co-construct knowledge in real time.
Dede’s use of the terms intelligence augmentation and partner further reveals the humanist architecture of his position.⁴ “AI is your partner,” he says, “and you’re stronger because you’re working together.” This metaphor is appealing, but it obscures the deeper ontological stakes. The language of partnership implies two stable agents working in tandem—AI supporting, the human directing. But this denies the extent to which cognition in AI-mediated environments is emergent, recursive, and systemically entangled. From a posthumanist perspective, we are not simply “stronger” with AI; we are different. The question is not how to collaborate with AI as it exists, but how to design systems where meaning-making itself is co-constructed. Here, co-emergence with AI is not the preservation of the human but a reconfiguration of the epistemic field
As someone working at the intersection of education, cognitive systems, and posthumanist theory, I find myself both aligned with and cautious of this framing. I support the pushback against instrumentalist models of automation and efficiency. But I resist the underlying premise of augmentation when it assumes a stable subject whose agency is simply extended or "boosted" by AI—rather than fundamentally transformed through its entanglement with machinic systems.
From Augmentation to Entanglement
In this respect, the language of "partnership" and "support" often underplays the deeper system dynamics at work—specifically, the emergence of the AI-human relation as a distributed cognitive system. What we are seeing is not simply a new tool in the hands of the learner or teacher, but a shift in the epistemic conditions under which learning takes place. This shift radicalizes what distributed cognition has meant in educational theory: it no longer refers only to collaboration across human minds or external artifacts, but to hybrid systems in which agency, intention, and interpretation are co-constructed across human and nonhuman actors.⁵
This shift, I would argue, marks a move from a humanist to a posthumanist epistemology. In a humanist framework, the boundaries of the subject are secure, cognition is internal, and learning is understood as an act of transfer or mastery. In a posthumanist frame, cognition is emergent, relational, and enacted within sociotechnical systems that exceed individual control or comprehension. AI doesn't merely augment our ability to think; it changes what thinking is, what counts as knowledge, and who (or what) gets to participate in its construction.
Personalization and the Politics of Agency
One of the most influential promises of AI in education is the promise of personalization. At its best, personalization is framed as a corrective to one-size-fits-all pedagogy: it allows instruction to adapt to individual learners, matching pace, content, and support to a student’s unique profile. In his public work, Dede has been a strong and much-needed critic of the marketing boosterism that surrounds this narrative—pushing back against reductive models that treat personalization as a mechanical process of optimizing content delivery. On this point, I am fully aligned with him. We share a commitment to rejecting the instrumental logic that equates learning with efficiency.
I also want to clarify that my argument is not opposed to the use of AI in education. I support and actively advocate for the thoughtful integration of AI into learning environments. But what I oppose—what must be critically examined—are the epistemological frames through which that integration takes place. Too often, personalization is imagined as a unidirectional system in which AI detects, diagnoses, and delivers, while the learner passively receives the appropriate intervention.⁶ Even in more nuanced versions of this model, the structure remains asymmetrical: AI adapts, the learner is adapted to.
This is where the limits of the humanist model begin to emerge. What is missing is a view of personalization not as optimization, but as dialogic and epistemic relation—one in which the learner has not only needs but voice, not only deficits but intentions. In this alternative framing, personalization becomes a space of negotiation, where students participate in shaping the trajectory of knowledge as it emerges in tandem with the system.
This is not a semantic distinction. It marks a deeper epistemological shift—from personalization as delivery to personalization as relation. In the dominant model, adaptation becomes a proxy for care, and accuracy a proxy for understanding. But this logic closes down movement. It forecloses struggle, hesitation, or refusal—those very disruptions that make learning possible. In such a system, even the promise of individualization becomes a mechanism of containment. Optimization becomes a form of epistemic closure.Dede rightly critiques personalization schemes that frame AI as a replacement for teacherly insight. Yet his own framing of personalization often leans on adaptive instruction that responds to learner needs but stops short of recognizing student agency as a force in shaping the epistemic architecture of the system itself. The model remains asymmetrical: AI adapts, the learner receives. Missing here is a view of personalization not as optimization but as dialogic space-making—a site in which learners negotiate, resist, and reconfigure how knowledge emerges in tandem with the system.⁷
This is not a semantic distinction. It marks a shift from personalization as delivery to personalization as a relational and epistemic act. Within this shift, student agency is no longer reactive but generative. It is precisely the insistence on adaptive accuracy that forecloses epistemic movement. In this sense, optimization becomes a form of closure.
Rethinking the Role of the Teacher: From Epistemic Authority to Relational Stewardship
The humanist frame that underpins much of the current discourse on AI in education—particularly the language of augmentation and partnership—retains a notion of human autonomy that is ultimately misleading. In aiming to preserve the sovereignty of the human thinker, it reasserts a bounded, self-contained subject who exercises control over tools, systems, and learning outcomes. Dede’s model, which emphasizes the irreplaceability of human judgment, fits squarely within this tradition. And while I share his concern about protecting space for judgment, I argue that this framing no longer maps onto the realities of AI-human cognition.
Within AI-mediated systems, cognition does not unfold within such clear separations. Agency is not a possession but a relational effect—emerging from patterned exchanges between human and machine, learner and interface, teacher and system. Ironically, it is the insistence on preserving classical autonomy that may undermine human agency as a meaningful force within this ecology.⁸ By treating the human as sovereign, it obscures the ways agency must now be distributed, negotiated, and reconfigured across the entire learning system.
This shift in perspective requires a corresponding rethinking of the teacher’s role. While Dede rightly emphasizes the importance of human judgment, his framing risks re-centering the teacher as epistemic authority: a figure who interprets, filters, and validates meaning on behalf of the learner. This move, though pedagogically well-intentioned, subtly reinstates a teacher-centered model of instruction—one rooted in transmission logics rather than relational emergence.
By contrast, a posthumanist pedagogy shifts the teacher’s role from that of epistemic gatekeeper to that of relational steward. The teacher does not direct knowledge, but orients the system—curating its ethical horizon, shaping its purpose, and cultivating the conditions under which cognition can emerge dialogically. Their task is not to ensure correct outcomes, but to help stabilize just enough structure for the system to evolve—without determining its trajectory in advance.
Even the oft-repeated claim that AI can “free up teachers” to focus on relational or creative work must be viewed with caution. It presumes that the educational system will recognize—and make space for—the unpredictability and interruption that meaningful pedagogical relations require. But as Gert Biesta argues, education is not merely a process of alignment or delivery.⁹ It is a space of intentional unpredictability—where subjectivity is formed through disruption and encounter, not simply input and output.
In practice, however, AI-driven EdTech often accelerates the very logic it purports to resist. It embeds educational processes more deeply within systems of managerial rationality—systems that privilege measurability, efficiency, and legibility. These systems render pedagogical relations illegible, or worse, irrelevant, within frameworks of reporting, accountability, and automated personalization. What begins as a call to deepen the relational dimensions of teaching can easily become a justification for automating everything else.
To move beyond this trap, we need a different epistemological frame—one that does not treat unpredictability as a problem to be managed, but as a constitutive condition of learning itself. This is where posthumanist pedagogy begins to shift the terms. Rather than assuming discrete actors (teacher, student, AI) operating within fixed roles, it sees cognition and relation as emergent properties of complex systems. The goal is not to delegate judgment to humans while assigning reckoning to machines, but to design systems capable of sustaining epistemic openness, relational disruption, and non-linear co-emergence. This means rethinking not just teaching or technology in isolation, but the entire architecture of learning—what I’ve elsewhere called the cognitive intraface: the space where human and machinic processes entangle, and where knowledge is not delivered, but negotiated.¹⁰
Dialogic Cognition in AI-Human Systems
If, as I’ve argued, we need to move beyond the humanist model of the teacher as epistemic authority and toward a view of the teacher as relational steward, then we must also reconsider how learning itself is conceptualized in AI-mediated systems. What replaces the transmission model cannot simply be a more interactive or adaptive version of instructional delivery. It must be a fundamentally different account of how knowledge emerges. This brings us to the concept of dialogic cognition—a framework that understands learning not as the acquisition of content, but as a co-constructed, relational process shaped by ongoing interaction. It is here that the limitations of augmentation become most visible: not only does the partnership model retain a fixed view of the human subject, but it also fails to account for how cognition itself unfolds through dialogue, tension, and recursive exchange within AI-human systems.¹¹
Much of the educational discourse around AI—especially in EdTech—remains oriented around one-way exchanges: systems that provide answers, recommend content, or automate feedback. Even when these systems claim to be interactive, they are rarely dialogic in any meaningful sense. They simulate conversation, but not cognition. They personalize, but they do not listen. What is missing is not just interactivity, but a model of thought that understands cognition as a dialogic process—one in which meaning does not preexist the exchange but emerges through it.¹²
Dialogic cognition is not simply about dialogue as a format; it is about dialogue as an epistemology. It understands thinking not as a linear progression toward truth, but as a recursive, relational movement shaped by difference, delay, and disruption. In human terms, this is familiar: we learn through dialogue not because another person gives us the right answer, but because the friction of the exchange prompts us to revise, clarify, and reflect. It is the tension—the moment of not-knowing—that catalyzes learning.
If we take this seriously, it has implications not only for pedagogy but for how we imagine and design AI systems themselves. Rather than building AI to deliver answers, we might design it to pose generative questions. Rather than providing feedback as correction, it might offer reflective counterpoints that surface assumptions. Rather than treating AI as a tool to be used, we might understand it as a participant in the cognitive ecology—a presence that shapes, constrains, and potentially expands what can be thought in a given context.
I refer to the site of this interaction as the cognitive intraface: the entangled threshold where human and machinic processes meet, not as external counterparts but as interwoven participants in epistemic activity. Within this space, cognition is no longer housed in the user or embedded in the system; it emerges from the recursive coupling of the two. Thought, in this model, is not transmitted or retrieved—it is co-constructed in situ.
To support dialogic cognition at the intraface, AI systems must be designed around a set of principles that differ from those guiding personalization, delivery, or optimization. These include:
- Metacognition: Systems must help users reflect not just on what they know, but on how they are knowing—surfacing uncertainty, implicit assumptions, and epistemic strategies.
- Reflexivity: AI must signal the contours of its own epistemic positioning—not as sentient, but as limited, heuristic, and historically trained.
- Reciprocity: Dialogue must be mutually responsive. The system must not merely wait to be prompted but adjust based on the unfolding logic of the user’s thought.
These are not just interface features. They are epistemological commitments. Without them, AI will continue to simulate interactivity while preserving the structure of delivery. Dialogic cognition, by contrast, insists that learning is not the result of accurate adaptation, but of epistemic friction sustained long enough to produce movement. It is not speed or certainty that makes thinking possible—but hesitation, relational tension, and the unfolding of inquiry across difference.
The Techno-Educational Matrix: AI’s Epistemological Frame Problem
The public discourse around AI in education tends to focus on model behavior, system accuracy, or the ethical boundaries of use. But these surface-level concerns often mask a deeper issue: the epistemological infrastructure within which those systems are imagined and implemented. Behind nearly every conversation about AI’s role in learning—whether celebratory or cautionary—is a shared operating assumption: that learning is something to be delivered, measured, and improved. That assumption is not neutral. It is the product of what I have elsewhere called the techno-educational matrix—a regime of thought in which cognition is treated as input-output processing and pedagogy as scalable optimization.
Within this matrix, AI is never just a tool; it is a symptom. A symptom of the deeper managerial rationality that has long governed how educational value is defined: not through emergence, ambiguity, or transformation, but through compliance, visibility, and control. It is no coincidence that EdTech products are typically built not around pedagogical complexity but around the logic of procurement, standardization, and user analytics. The result is a suite of systems that, even when adorned with the language of personalization or partnership, reinforce transmissive models of learning and suppress dialogic, disruptive, or unquantifiable forms of understanding.
This is the context in which Dede’s augmentation model must be understood. While well-intentioned—and grounded in a desire to preserve human judgment—it still operates within the very matrix it seeks to reform. By assuming that judgment and creativity can be preserved atop a base layer of AI-driven optimization, it unintentionally accepts the vertical logic of control it otherwise resists. What’s needed is not a better tiering of responsibilities between humans and machines, but a reimagining of the epistemic architecture altogether: one that refuses the delivery model and instead builds systems for epistemic movement, relational inquiry, and ontological emergence.
Conclusion: Designing for Epistemic Openness
This essay has argued that the dominant humanist framing of AI in education—especially through the language of augmentation, personalization, and partnership—remains epistemologically insufficient. While thinkers like Chris Dede rightly challenge automation-centered models, their efforts to preserve human judgment often rest on assumptions of cognitive sovereignty and bounded subjectivity that no longer hold.¹³ AI is not simply a tool to support human reasoning. It is part of a shifting epistemic ecology in which agency, knowledge, and learning emerge through entangled interaction.¹⁴
Throughout, I’ve proposed a posthumanist alternative—one that sees the teacher not as epistemic authority but as relational steward, personalization not as optimization but as dialogic negotiation, and AI not as partner or proxy but as participant in a system of distributed cognition. At the center of this vision is the cognitive intraface, a site where meaning is co-constructed, contested, and reflexively re-formed through ongoing AI-human exchange.
Yet these alternatives cannot be implemented in a vacuum. They must be understood in relation to the techno-educational matrix—the broader epistemic regime that continues to shape what counts as knowledge, how learning is defined, and who gets to speak within educational systems. Without confronting this matrix, even the most well-intentioned models risk being absorbed into a logic of optimization, compliance, and epistemic control.¹⁵
The shift I’ve called for here is not merely technical or pedagogical. It is epistemological. It demands that we not only redesign AI systems, but also reshape the conditions of reception: how AI is talked about, taught with, and held accountable within institutional and pedagogical cultures. It asks us to resist the closure of platform logic—the performance of knowing, the compression of nuance, the framing of critique as brand—and to design for epistemic openness instead: slow, recursive, dialogic, and shared.¹⁶
Footnotes
¹ See Bakhtin (1981) for the foundational theory of dialogism, in which meaning emerges through interaction and difference, not isolated thought. This essay extends his epistemology to AI-human cognition.
² Peters and Jandrić (2019) introduce the idea of postdigital knowledge ecologies—nonlinear, socio-technical environments that contextualize the techno-educational matrix described in this essay.
³ Dede (2023) draws a distinction between “reckoning” and “judgment,” preserving human roles in ethical discernment.
⁴ Dede (2024) reiterates his human-centered framing of creativity and partnership in his Silver Lining for Learning episode, which this essay reads as bounded by a humanist subject model.
⁵ Earlier models of distributed agency—beginning with Vygotsky’s theory of semiotic mediation and extended by scholars such as Wertsch (1998), Pea (1993), and Hutchins (1995)—conceive of cognition as socially and materially distributed across people, tools, and cultural contexts. These frameworks disrupt individualist models of mind by emphasizing interaction and mediation, but they often retain a functionalist view of tools as instruments for human use. This essay extends that lineage by adopting a posthumanist lens in which agency is not only distributed but co-emergent, entangled within sociotechnical systems that reshape cognition itself.
⁶ A growing body of critique has questioned the overuse and conceptual vagueness of “personalization” in EdTech. Scholars note that many so-called personalized systems reduce learning to the automated matching of content to inferred needs—obscuring asymmetrical power dynamics while reinforcing a delivery model of education. Personalization, in this context, often functions more as a rhetorical device than a meaningful pedagogical design.
⁷ Wegerif (2013) develops the concept of dialogic space as a pedagogical and epistemological alternative to monologic instruction. Drawing on Bakhtin and Vygotsky, he argues that digital technologies can support dialogic learning only if they are intentionally designed to sustain open-ended, co-constructive inquiry—rather than reinforcing the closed, outcome-driven logic typical of EdTech.
⁸ Suchman (2007) provides the foundational theory of situated action, showing how meaning and intention emerge through interaction rather than preexisting plans.
⁹ Biesta (2013) calls for educational practice that welcomes interruption and unpredictability as constitutive of subject formation—a view this essay aligns with against optimization logic.
¹⁰ Wertsch (1998) frames cognition as mediated action, not internal content—supporting the cognitive intraface as a site of emergent meaning.
¹¹ Hayles (2017) explores the cognitive nonconscious as a layer of distributed awareness that reframes cognition beyond conscious or individual control—relevant to the posthumanist cognition described here.
¹² Wegerif (2013) and Mercer (2019) both argue that digital technologies must support dialogic spaces—where meaning is co-constructed through interaction, rather than delivered. This essay builds on their work by emphasizing not just the conditions for dialogue, but the epistemic implications of designing AI systems that think dialogically.
¹³ See Hayles (1999) for a genealogical critique of the liberal humanist subject and its entanglement with informational and cybernetic logics. This essay continues that project into the context of AI education.
¹⁴ Hayles (2025) articulates a posthumanist view of cognition as symbiotic and distributed, providing the ontological basis for the epistemic framework advanced here.
¹⁵ Peters (2017) critiques the collapse of epistemic authority in the post-truth condition, a concern that is extended here to the algorithmic regimes of educational AI.
¹⁶ Hayles (2012) develops the notion of technogenesis, arguing that human cognition co-evolves with media technologies—a claim this essay builds upon in relation to AI.
Works Cited.
Bakhtin, Mikhail. 1981. The Dialogic Imagination: Four Essays. Edited by Michael Holquist, translated by Caryl Emerson and Michael Holquist. Austin: University of Texas Press.
Biesta, Gert. 2013. The Beautiful Risk of Education. Boulder, CO: Paradigm Publishers.
Bulger, Monica. 2016. Personalized Learning: The Conversations We’re Not Having. Data & Society Research Institute.
Dede, Chris. 2023. “Educating in a World of Artificial Intelligence.” Harvard EdCast. Harvard Graduate School of Education, February 23, 2023. https://www.gse.harvard.edu/ideas/edcast/23/02/educating-world-artificial-intelligence.
Dede, Chris. 2024. Silver Lining for Learning: Episode 158—Creativity and Generative AI. YouTube video, 1:02:44. Posted April 20, 2024. https://www.youtube.com/watch?v=HrNY_FFUQpw&t=32s.
Hayles, N. Katherine. Bacteria to AI: Human Futures with Our Nonhuman Symbionts. Chicago: University of Chicago Press, 2025. https://doi.org/10.7208/chicago/9780226837468.001.0001
Hayles, N. Katherine. 2017. Unthought: The Power of the Cognitive Nonconscious. Chicago: University of Chicago Press.
Hayles, N. Katherine. 2012. How We Think: Digital Media and Contemporary Technogenesis. Chicago: University of Chicago Press.
Hayles, N. Katherine. 1999. How We Became Posthuman: Virtual Bodies in Cybernetics, Literature, and Informatics. Chicago: University of Chicago Press.
Hutchins, Edwin. 1995. Cognition in the Wild. Cambridge, MA: MIT Press.
Mercer, Neil, Sara Hennessy, and Paul Warwick. “Dialogue, Thinking Together and Digital Technology in the Classroom: Some Educational Implications of a Continuing Line of Inquiry.” International Journal of Educational Research 97 (2019): 187–199.
Pea, Roy D. 1993. “Practices of Distributed Intelligence and Designs for Education.” In Distributed Cognitions: Psychological and Educational Considerations, edited by G. Salomon, 47–87. Cambridge: Cambridge University Press.
Peters, Michael A. 2017. “Education in a Post-Truth World.” Educational Philosophy and Theory 49 (6): 563–566. https://doi.org/10.1080/00131857.2016.1264114.
Peters, Michael A., and Petar Jandrić. 2019. “Postdigital Knowledge Ecologies.” In Postdigital Science and Education, edited by Michael A. Peters, Petar Jandrić, and Alexander J. Means, 1–20. Singapore: Springer. https://doi.org/10.1007/978-981-32-9340-7_1.
Selwyn, Neil. 2016. “Minding Our Language: Why Education and Technology is Full of Bullshit… and What Might Be Done About It.” Learning, Media and Technology 41(3): 437–443.
Suchman, Lucy. 2007. Human-Machine Reconfigurations: Plans and Situated Actions. 2nd ed. Cambridge: Cambridge University Press.
van Dijck, José, Poell, Thomas, and de Waal, Martijn. 2018. The Platform Society: Public Values in a Connective World. Oxford University Press.
Vygotsky, L. S. 1978. Mind in Society: The Development of Higher Psychological Processes. Edited by Michael Cole et al. Cambridge, MA: Harvard University Press.
Watters, Audrey. 2017. “The Weaponization of Education Data.” In The Monsters of Education Technology 2.
Wegerif, Rupert. Dialogic: Education for the Internet Age. London: Routledge, 2013.
Wegerif, Rupert. “Dialogic Theory and Technology-Mediated Learning.” In The Routledge International Handbook of Research on Dialogic Education, 2020.
Wertsch, James V. 1998. Mind as Action. New York: Oxford University Press.